As a school district leader, you are tasked with making decisions that impact student outcomes. One method of analyzing data utilizes predictive analytics. At ECRA, many of our products harness the power of predictive analytics to help school district leaders set goals, make decisions, and assess the impact of programming/interventions.
In this article, we will provide an overview of predictive analytics, including its definition, key concepts, and applications in education. We will also discuss some of the challenges associated with predictive analytics and provide some best practices for implementing this technology in a school district setting.
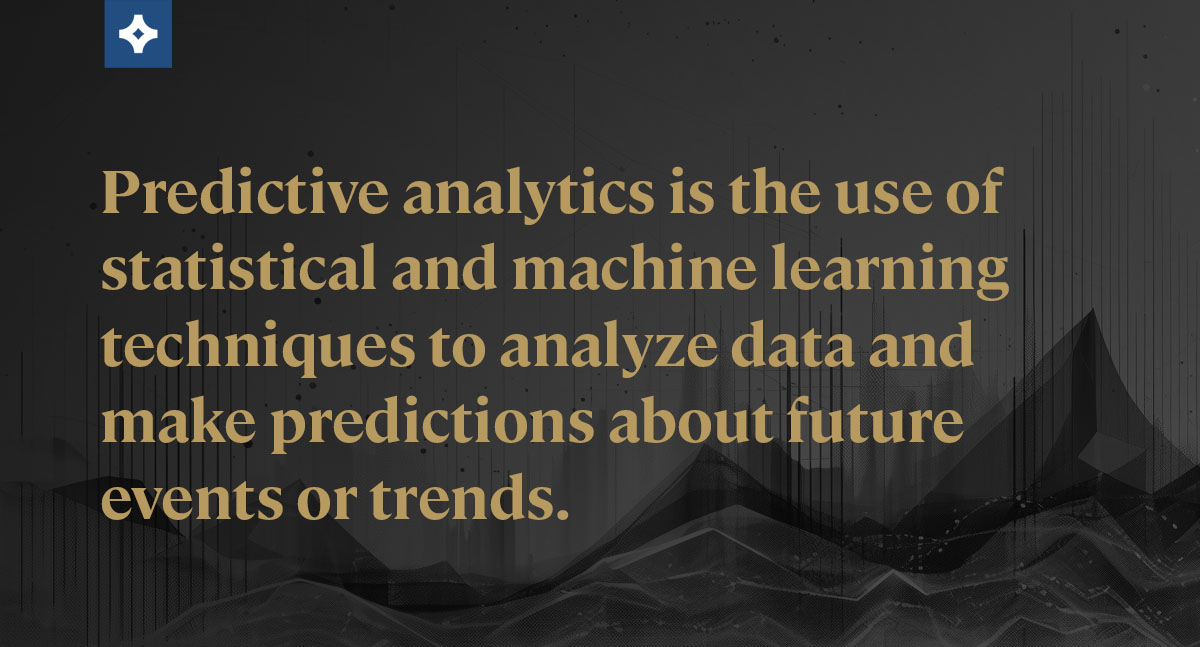
What is Predictive Analytics?
Predictive analytics is the use of statistical and machine learning techniques to analyze data and make predictions about future events or trends. It involves the use of historical data to develop models that can be used to identify patterns and make predictions based on new data.
In the K-12 education sector, predictive analytics is used to identify students who are at risk of falling behind. Oftentimes, this is accomplished by analyzing a wide range of data points, including attendance records, grades, disciplinary records, demographic information, and more.
Predictive Analytic Use Outside of Education
Sometimes, to understand how useful and widespread the use of predictive analytics is, understanding how much it is embedded in our everyday life can be helpful. Predictive analytics has numerous applications outside of the education sector. It is used in a wide range of industries and fields, including healthcare, finance, marketing, weather, and transportation.
Healthcare
One area where predictive analytics has been particularly useful is in healthcare. Predictive analytics can be used to analyze patient data to identify individuals who are at risk of developing certain diseases or conditions. This can help healthcare providers to take proactive steps to prevent or treat these conditions, improving patient outcomes and reducing healthcare costs. For example, predictive analytics can be used to identify patients who are at risk of developing diabetes, allowing healthcare providers to offer targeted interventions such as lifestyle coaching and medication to prevent the onset of the disease.
Finance
Another area where predictive analytics is widely used is in finance. Predictive analytics can be used to analyze financial data to identify trends and patterns that can help companies to make better business decisions. Banks use predictive analytics to analyze customer data to identify individuals who are at risk of defaulting on loans, allowing them to take proactive steps to prevent these defaults and reduce financial losses.
Weather
Each day, when you are deciding how to dress appropriately for the day, you’ve harnessed the power of predictive analytics. It is widely used in weather forecasting. Predictive analytics can be used to analyze data from various sources, including satellites, weather stations, and sensors, to generate accurate and timely weather forecasts. These forecasts help individuals and organizations to prepare for severe weather events, reducing the risk of property damage, injury, and loss of life. Not to mention, they let you know if you could benefit from bringing an umbrella on your way out the door.
Predictive analytics has numerous applications outside of education, and is used in a wide range of industries and fields to improve outcomes, make lifestyle choices, and reduce risk. It has the potential to transform the way that we use data to make decisions and solve complex problems. Knowing its use in other industries may help you, as a district leader, realize that widespread use of predictive analytics exists and truly should be used as a powerful reference point when making decisions at your school district or when setting goals.
Key Concepts in Predictive Analytics
There are several key concepts that are important to understand when it comes to predictive analytics, including:
- Data Preparation: Before predictive analytics can be used, data must be collected, cleaned, and prepared for analysis. This involves identifying relevant data sources, cleaning and transforming the data, and selecting appropriate variables for analysis.
- Statistical Modeling: Predictive analytics involves the use of statistical models to identify patterns and trends in the data. These models can take many different forms, including linear regression, decision trees, and neural networks.
- Machine Learning: Machine learning is a subfield of artificial intelligence that involves developing algorithms that can learn from data. Machine learning algorithms can be used to build predictive models that can make predictions about future outcomes.
- Validation: It is important to validate predictive models to ensure that they are accurate and reliable. This involves testing the model on new data to see how well it performs.
Applications of Predictive Analytics in Education
There are several applications of predictive analytics in education. Some of the most common include:
- Identifying At-Risk Students: Predictive analytics can be used to identify students who are at risk of falling behind or dropping out. This can be done by analyzing a wide range of data points, including attendance records, grades, disciplinary records, and demographic information.
- Personalizing Learning: Predictive analytics can be used to develop personalized learning plans for students based on their individual needs and learning styles. This can help to improve student outcomes and reduce dropout rates.
- Improving Resource Allocation: Predictive analytics can be used to identify areas where resources, such as teachers or instructional materials, are needed the most. This can help to ensure that resources are allocated in an efficient and effective manner.
Benefits of Predictive Analytics in Education
While the computations and analysis involved in predictive analytics are difficult for many districts to accomplish on their own, once achieved, they make data analysis and use much simpler and easier than nearly all previous practices. The reason is that they help to sift through the morass of data, and they project statistically significant outcomes for students, cohorts, schools, and districts. If the desired outcomes do not match the predicted outcomes, then decisions for educators come into focus very quickly, and they can design programs and interventions that will have an impact on the predicted outcomes.
No longer do educators have to perform annual autopsies of old data and guess at what programs will work best on new sets of students. The use of predictive analytics makes decision-making, goal setting, and program analysis simple, measurable, and transparent.
Improved Student Outcomes
Predictive analytics has the potential to significantly improve student outcomes by identifying those who may be at risk of academic or behavioral issues. By analyzing data on student performance and behavior, school district leaders can identify patterns that suggest a need for intervention, allowing for more timely, targeted and personalized support. Over time, the data collected through predictive analytics can also help educators track student progress and adjust interventions as needed, leading to more effective support that can improve outcomes for individual students and entire classes. Ultimately, using predictive analytics to identify which students need additional support and providing that support in a timely and meaningful manner, school district leaders can help more students achieve academic and behavioral success.
Efficient Resource Allocation
With limited resources available, school district leaders must make strategic decisions about where to allocate funding, staffing, and materials to best support student outcomes. Predictive analytics help inform these decisions by identifying areas where resources are most needed and which interventions are most likely to have a positive impact. By taking a data-driven approach to resource allocation, school district leaders will make more informed decisions about where to invest their resources and which programs and initiatives are most likely to have the greatest impact on student outcomes. Over time, this leads to more efficient and effective use of resources and improved outcomes for students across the district.
Data-Driven Decision Making
Data can be a powerful tool for school district leaders looking to make informed decisions that will have a positive impact on student outcomes. Building a data culture in a school and district can be difficult due to all of the data available, difficulties connecting data, and then analyzing data. The use of predictive analytics actually makes data analysis simpler and easier to act upon. Predictive analytics help school district leaders identify trends and patterns that may not be immediately obvious, allowing them to make more informed decisions about everything from student placement to curriculum development. By using data to inform decision making, school district leaders can also be more confident that the choices they make will have a positive impact on students, leading to better outcomes and increased accountability. Ultimately, by taking a data-driven approach to decision making, school district leaders can uncover new insights and strategies that can improve outcomes for students in ways that might not have been possible without data.
Predictive Analytics Techniques in Education
Here are a few common techniques used in predictive analytics for education:
- Regression Analysis: This technique is used to identify relationships between variables and can be used to predict outcomes based on historical data. In education, regression analysis may be used to predict student performance based on factors such as previous academic achievement, attendance, and demographic information.
- Decision Trees: Decision trees are a visualization tool used to identify decision points and potential outcomes based on data. In education, decision trees may be used to identify factors that contribute to student success or failure and help educators make data-driven decisions about interventions and support.
- Neural Networks: Neural networks are a type of machine learning algorithm that can be used to identify patterns in data and predict future outcomes. In education, neural networks may be used to predict student performance based on factors such as behavior, attendance, and academic history.
- Clustering: Clustering is a technique used to group data points based on similarities. In education, clustering may be used to group students with similar characteristics or academic histories, allowing educators to provide targeted support and interventions.
Challenges and Best Practices in Education
While predictive analytics has the potential to significantly impact goal setting and decision making at a school district, there are several challenges associated with implementing this technology. Below are a few key challenges:
- Data Quality: Predictive analytics relies on high-quality data. If the data is incomplete or inaccurate, the predictions made by predictive models may not be reliable.
- Privacy Concerns: Predictive analytics involves the use of sensitive student data. It is important to ensure that this data is protected and used in a responsible manner.
- Ethical Considerations: Predictive analytics can raise ethical concerns, particularly when it comes to issues such as student privacy, bias, and fairness.
To ensure that predictive analytics is used in a responsible and effective manner, it is important to follow some best practices, including:
- Develop a Clear Plan: Before implementing predictive analytics, it is important to develop a clear plan that outlines the goals and objectives of the project, the data sources that will be used, the models that will be developed, and how the results will be used.
- Use High-Quality Data: To ensure that predictive models are accurate and reliable, it is important to use high-quality data that is complete, accurate, and up-to-date. This may require cleaning and transforming the data before it can be used.
- Ensure Data Privacy: It is important to ensure that student data is protected and used in a responsible manner. This may require implementing data security measures, such as encryption and access controls, and obtaining informed consent from students and their families.
- Address Ethical Concerns: Predictive analytics can raise ethical concerns, such as issues of bias and fairness. It is important to address these concerns by ensuring that models are developed in a transparent and accountable manner, and by monitoring their performance over time.
- Evaluate and Improve: Predictive analytics is an iterative process that requires ongoing evaluation and improvement. It is important to regularly evaluate the performance of predictive models and to make adjustments as needed to ensure that they remain accurate and reliable.
FAQs about Predictive Analytics
What kind of data is used in predictive analytics in education?
Predictive analytics in education can use various types of data such as attendance records, grades, disciplinary records, demographic information, and behavioral data.
Can predictive analytics be used to personalize learning?
Yes, predictive analytics can be used to develop personalized learning plans for students based on their individual needs and learning styles.
Is student data protected when using predictive analytics in education?
It is important to ensure that student data is protected and used in a responsible manner. This may require implementing data security measures, such as encryption and access controls, and obtaining informed consent from students and their families.
How can schools address ethical concerns with predictive analytics?
Schools may address ethical concerns by ensuring that models are developed in a transparent and accountable manner, and by monitoring their performance over time. It is also important to involve stakeholders in the development and implementation of predictive analytics projects.
How can schools ensure that predictive analytics is used effectively?
To ensure that predictive analytics is used effectively, schools should develop a clear plan, use high-quality data, ensure data privacy, address ethical concerns, and evaluate and improve the performance of predictive models over time.
What is machine learning?
Machine learning is a subfield of artificial intelligence that involves the use of algorithms and statistical models to enable machines to learn from data, without being explicitly programmed. In other words, machine learning enables computers to automatically identify patterns and relationships in data, and to use this information to make predictions or take actions without the need for human intervention. Machine learning is widely used in a variety of applications, including image and speech recognition, natural language processing, and predictive analytics.
How do ECRA’s Solutions and Products Use Predictive Analytics?
Predictive analytics is a powerful tool that can help school districts identify at-risk students, personalize learning, and allocate resources more effectively. However, it is important to use this technology in a responsible and ethical manner, taking into account the concerns of stakeholders such as students, families, and educators.
ECRA’s School Improvement solution harnesses the power of predictive analytics. This web-based application gives school districts an actionable data and analytics solution designed to measure and improve student outcomes. Your use of the most sophisticated predictive analytics in the K-12 education marketplace empowers you to measure student growth, set actionable goals, and monitor programming for academic return on investment (ROI). If your school district would like to see how, please connect with us to schedule a demo or just have a conversation. Our products are designed to help maximize student success.