Leveraging predictive models to measure learning loss and growth recovery during COVID-19 school closures
Given vast school closures during the spring and fall of 2020, an important question remains:
“How do we measure learning loss
and growth recovery of students?”
Measuring learning loss requires that we recognize that student growth is personal in the sense that every individual student’s learning trajectory leading up to the pandemic was different.
The measurement strategy is to create a predictive model capable of generating controls for individual students and groups of students in order to compare current learning trajectories to projected learning trajectories that likely would have occurred if learning was uninterrupted by COVID-19. Addressing the counterfactual of what would have likely happened to individual students had the COVID-19 pandemic never happened is the key to measuring learning loss and growth recovery.
Generating accurate projections as to what likely would have happened without the pandemic requires that one create separate learning trajectory models for each and every student within a school district.
Predictive Framework for Measuring Learning Loss
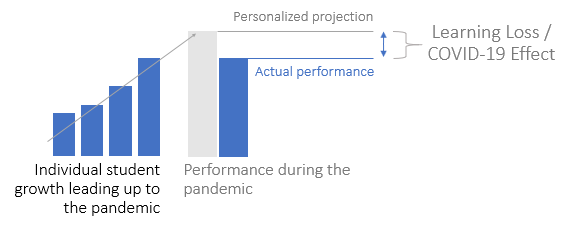
Using a projected versus actual framework allows data scientists to express the difference between a student’s projected learning trajectory and their actual learning trajectory as an effect size. Expressing learning loss and growth recovery as an effect size has the advantage of leveraging decades of research on educational effect sizes and allows individual student learning loss to be averaged across tests, subjects, and grades, or any other variables of interest – allowing for ease of use and actionable results through thresholds.
While numerous neural network and regression-based machine learning models can be trained to build a predictive model, they all anchor to a common conceptual framework. Specifically, historical student data is used to train a predictive model that learns individual student growth rates and the growth rates of similar students. Once the model is trained, projected learning trajectories can be generated that are personalized to each and every student.
Machine Learning Framework to Train a Learning Trajectory Model
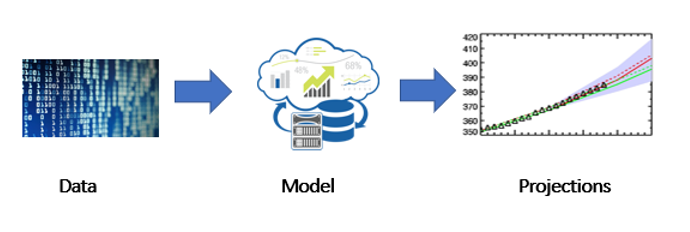
The most difficult part of training a learning trajectory model is choosing which types of data you will allow or not allow the model to consider as it learns. While it is tempting to provide a machine learning model as much data as you have, this is not the correct approach. The most widely misunderstood cliché in machine learning is the notion that more data is always better. The decision for which kinds of data you will feed the machine learning model has political, policy, and statistical implications.
If you would like to talk further on how to leverage predictive models and machine learning to measure learning loss and growth recovery, contact me on twitter @jlgatta. A non-technical video related to learning loss and growth recovery can also be found below: